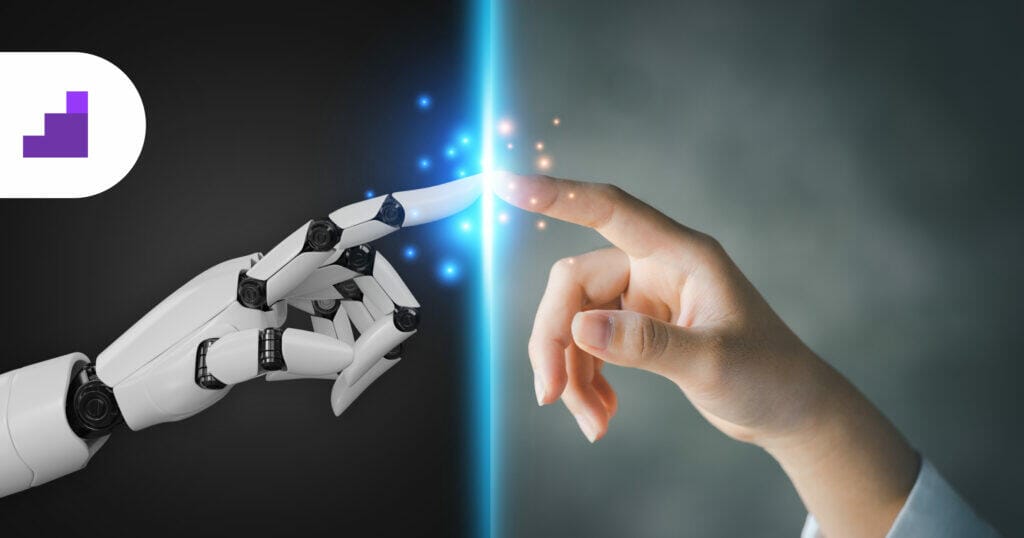
The promise of AI is a huge one. Predictions can be made with unprecedented scale and accuracy. Patterns in languages can offer dimensions in data that are otherwise unseen. Supervised and non-supervised deep learning techniques can result in mission critical actions or recommendations. Let’s dig a bit deeper into what this can mean in practical terms.
The Existence of AI
AI is not human and would not exist or be able to prosper but for the human factor.
Each of our individual brains has 86 billion organic neurons. While we are not programmable machines, per se, humans have enormous capacity for ingestion/organization of data points, for producing intelligence by mindful analyses of these data, for transforming what is learned into sound judgement, actions and for rendering accurate predictions. Now multiply a factor x 86 billion neurons by the total of everyone on your team.
The Value of AI
Despite impressions to the contrary, AI application use cases remain far and few between.
For AI to bring value to any cause, social, business or marketing problem, it has to be constructed by humans. Not just any humans, but humans who possess a deep understanding of the nature of the problem that needs to be solved, the history of the problem’s evolutions, the context of the problem, previously proven/disproven solutions, degrees of potential causes of the problems, all the data elements potentially relevant to machine learning inputs and more.
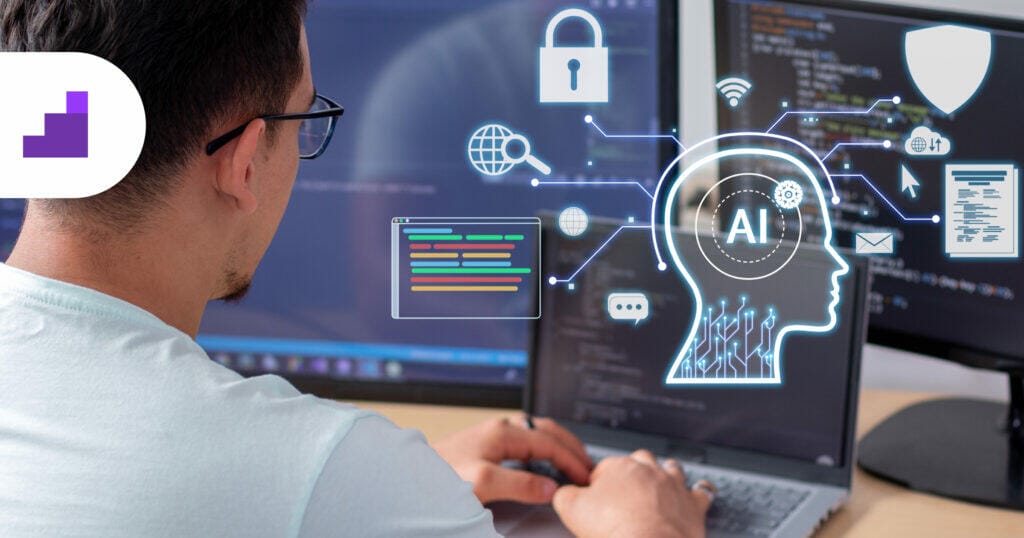
Machine Learning and AI
Machine Learning and AI has been successfully implemented for decades.
I am referring, for example, to individualized surveys that predict personality, social and competency attributes. Multiple regression techniques have been utilized for decades to uncover the hidden signals and patterns in the answers we give to the 1-1 surveys. The narratives/predictions that pop out of these models boast consistent accuracy rates in the high 90% range. Can AI do more? Yes, but I think there are important lessons from past successes to consider.
Real-World ‘True/False’ Accuracy
Most predictive models are excellent at isolating correlative, even causal predictors to all types of outcomes but not necessarily real-world ‘true/false’ accuracy.
This is a distinction with a difference. Predictive models are able to predict which features are predictive for any given result and not necessarily to the degree of acceptable True/False real-world accuracy. Predictive models tell us what is important and very often why it is important. To achieve better real-world accuracy, machine learning apps have been used to reprocess predictors and other relevant but untested data elements. Machine learning techniques can be used in this context to identify which combinations of data elements will result in highest accuracy levels.
Final thoughts
In practical terms, AI cannot progress to include more use cases without humans using their respective 86 billion neurons to architect, plan, test, and orchestrate all the paths forward. Whether it’s related to natural language or deep learning or neural models that improve real world accuracy, our individual organic neurons, not to mention our boundless empathies, can unite with machine learning techniques to make future innovations, algorithms/AI solutions viable.
The most successful solutions have been engineered in reverse. They started with the end purpose in mind, followed by the strategies, elements and steps required to achieve the desired outcome.
Send me a message or book a free 30-minute Intro meeting with me to get the conversation started!
Not sure what expertise you need? Our concierge is happy to discuss your needs and match you with the best suited consultant. On Cansulta, you can browse consultants by expertise, and connect with vetted professionals in Intro sessions FREE.